Real-Time Analytics Platforms: Choosing the Right Solution for Your Business Success
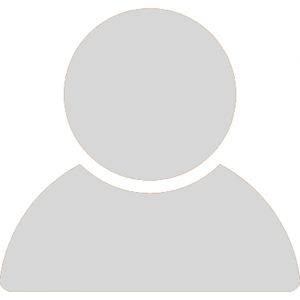
Audio : Listen to This Blog.
Businesses need to process and analyze data quickly to stay competitive. Data ingestion is a critical component of real-time analytics, allowing businesses to collect and process data as it is generated. Real-time analytics refers to a type of data analysis that focuses on delivering insights to end users in real time. Real-time analytics platforms enable organizations to gain immediate insights from their data, facilitating rapid decision-making and enhancing operational efficiency. As we move into 2024, the importance of real-time analytics continues to grow, with advancements in technology making these platforms more accessible and powerful. This article explores the essentials of real-time analytics platforms, key features to consider, and how to choose the right solution for your needs.
What is Real-Time Analytics?
Source: Rockset
Real-time analytics involves the immediate processing and analysis of data as it is generated, often through event processing. Real-time data analytics provides significant benefits, such as timely decision-making, user-facing analytics, and enhanced capabilities through real-time analytics databases. Unlike traditional analytics, which often relies on historical data and batch processing, real-time analytics allows businesses to gain instant insights and act on them without delay. This capability is crucial for various applications, including monitoring customer behavior, detecting fraud, optimizing supply chains, and improving user experiences.
Importance of Real-Time Analytics in 2024
The digital transformation of businesses across industries has led to an explosion of data generation. Analyzing data in various industries and use cases such as financial services, e-commerce, and healthcare is crucial for leveraging this data effectively. Leveraging this data effectively requires the ability to process and analyze it in real-time. In 2024, the following factors underscore the importance of real-time analytics:
- Enhanced Decision-Making: With real-time insights, businesses can make informed decisions quickly, adapting to market changes and customer needs more effectively. Real-time insights enable data-driven decision making, allowing businesses to base their strategies on the most current information available.
- Improved Customer Experiences: Real-time analytics enables personalized interactions and immediate responses to customer queries, enhancing satisfaction and loyalty.
- Operational Efficiency: By monitoring operations in real-time, organizations can identify and address issues promptly, reducing downtime and optimizing processes.
- Competitive Advantage: Businesses that can analyze and act on data faster than their competitors gain a significant edge in the market.
Key Features of Real-Time Analytics Platforms
Source: Qlik
When choosing a real-time analytics platform, it’s essential to consider several key features that can impact its effectiveness and suitability for your business needs:
- Data Integration: The ability to integrate data from various sources seamlessly, including stream processing, is crucial. Look for platforms that support multiple data types and formats, ensuring comprehensive data analysis.
- Scalability: As your business grows, so will your data. Choose a platform that can scale efficiently to handle increasing data volumes without compromising performance. Specialized hardware and software systems are vital in managing the growing quantities and diversity of data for real-time analytics applications.
- Low Latency: For true real-time analytics, low data latency is essential. The platform should process and deliver insights with minimal delay to enable prompt action.
- User-Friendly Interface: A platform with an intuitive interface makes it easier for users at all levels to navigate and utilize its features effectively.
- Advanced Analytics Capabilities: Look for platforms that offer advanced analytics features such as predictive analytics, machine learning integration, and anomaly detection.
- Security and Compliance: Ensure the platform adheres to industry standards for data security and compliance, protecting sensitive information and maintaining regulatory requirements.
- Customization and Flexibility: The ability to customize dashboards, reports, and analytics workflows to meet specific business needs is valuable.
- Cost-Effectiveness: Evaluate the total cost of ownership, including licensing, implementation, and maintenance costs, to ensure the platform fits within your budget.
Best Real-Time Analytics Platforms in 2024
1. Google Cloud Dataflow
Google Cloud Dataflow is a fully managed service for stream and batch data processing. It supports complex data processing workflows and integrates seamlessly with other Google Cloud services.
Key Features:
- Unified stream and batch processing
- Auto-scaling and dynamic workload balancing
- Integration with Google BigQuery for advanced analytics
- Real-time data pipelines and ETL processes
- Enables users to access data quickly from multiple sources for immediate analysis
Pros:
- High scalability and flexibility
- Strong integration with Google Cloud ecosystem
- Robust security features
Cons:
- Can be complex to set up and manage
- Higher costs for large-scale deployments
2. Apache Kafka
Apache Kafka is an open-source stream-processing platform designed for high-throughput, low-latency data streaming. It’s widely used for building real-time data pipelines and streaming applications.
Key Features:
- Distributed and fault-tolerant architecture
- High throughput for handling large volumes of data
- Real-time data streaming and processing
- Integration with various data storage and processing systems
- Native support for semi-structured data, enabling efficient real-time analytics without the need for ETL processes
Pros:
- Highly scalable and reliable
- Open-source and community-supported
- Versatile integration capabilities
Cons:
- Requires significant setup and maintenance effort
- Steeper learning curve for new users
3. Microsoft Azure Stream Analytics
Microsoft Azure Stream Analytics is a real-time analytics service designed for complex event processing and real-time data integration. It provides a simple, scalable, and cost-effective solution for real-time data analysis.
Key Features:
- Real-time data streaming and event streams processing
- Integration with various Azure services
- Easy-to-use query language similar to SQL
- Built-in machine learning and predictive analytics capabilities
Pros:
- Seamless integration with Azure ecosystem
- Scalable and flexible pricing models
- User-friendly interface
Cons:
- Limited to Azure cloud environment
- Potentially higher costs for extensive use
4. IBM Descriptive Analytics Tools
IBM offers a suite of analytics tools that leverage AI and machine learning to provide advanced insights. IBM Cognos Analytics, for instance, is a comprehensive business intelligence solution that supports data exploration, visualization, and reporting.
Key Features:
- Advanced analytics capabilities including AI integration and predictive analytics
- Strong data visualization and reporting tools
- Scalable and flexible architecture
- Robust security and compliance features
Pros:
- Integration with AI and machine learning
- Comprehensive data visualization tools
- Strong support and documentation
Cons:
- Can be costly for small to mid-sized businesses
- Requires technical expertise for full utilization
5. Amazon Kinesis
Amazon Kinesis is a suite of services designed for real-time data streaming and analytics. It offers capabilities for data ingestion, processing, and analysis in real-time.
Key Features:
- Real-time data ingestion and processing
- Integration with AWS services for data storage and analytics
- Scalability to handle large data volumes
- Stream processing with Kinesis Data Streams and Firehose
Pros:
- High scalability and flexibility
- Strong integration with AWS ecosystem
- Reliable and secure
Cons:
- Complex pricing structure
- Requires AWS knowledge for optimal use
- Choosing the Right Real-Time Analytics Platform
Choosing the Right Platform
Selecting the right real-time analytics platform involves careful consideration of your business needs, data requirements, and long-term goals. Here are some steps to guide your decision-making process:
1. Assess Your Business Needs
Identify the specific needs and goals of your business. Consider the types of data you collect, the volume of data, and the frequency at which you need insights. Understanding your requirements will help you choose a platform that aligns with your objectives.
2. Evaluate Integration Capabilities
Ensure the platform can integrate with your existing systems and data sources, providing seamless data integration for comprehensive analysis. Seamless integration is crucial for comprehensive data analysis and avoiding data silos.
3. Consider Scalability
Choose a platform that can scale with your business. As your data volume grows, the platform should be able to handle increased loads without compromising performance.
4. Prioritize User Experience
A user-friendly interface and intuitive design make it easier for your team to adopt and utilize the platform effectively. Consider platforms that offer customizable dashboards and easy-to-use tools.
5. Review Security and Compliance
Data security and compliance are critical considerations. Ensure the platform adheres to industry standards and provides robust security features to protect sensitive information.
6. Analyze Cost and ROI
Evaluate the total cost of ownership, including licensing, implementation, and maintenance costs. Consider the potential return on investment (ROI) from improved decision-making, operational efficiency, and enhanced customer experiences.
Future Trends in Real-Time Analytics
As we move further into 2024, several trends are shaping the landscape of real-time analytics:
1. AI and Machine Learning Integration
The integration of AI and machine learning with real-time analytics tools will continue to grow, providing more advanced insights and automating routine analysis tasks. These technologies enable predictive analytics, anomaly detection, and deeper data insights, transforming how businesses interpret and act on data.
2. Real-Time Data Processing
The demand for real-time data processing will increase, enabling businesses to make faster and more informed decisions. Minimizing data latency is crucial for real-time data processing, enabling businesses to respond to changes instantly. Real-time data processing helps in quickly detecting and responding to patterns in user behavior, providing personalized experiences and recommendations. Platforms will enhance their capabilities to handle and process data instantaneously, supporting applications that require immediate insights, such as fraud detection and dynamic pricing.
3. Enhanced Data Visualization
Improved data visualization techniques will make it easier for users to understand and interpret complex data sets. Innovations in visual analytics will allow for more interactive and intuitive representations of data, aiding in quicker and more accurate decision-making.
4. Self-Service Analytics
Self-service analytics tools will become more prevalent, allowing non-technical users to perform their own data analysis and create custom reports. This democratization of analytics empowers all employees to leverage data insights, fostering a data-driven culture within organizations.
5. Mobile Accessibility
Mobile-friendly analytics tools will become essential, allowing users to access insights and reports on the go. As remote work and mobile device usage increase, the ability to analyze data and make decisions from anywhere becomes crucial.
Implementing Real-Time Analytics in Your Business
Implementing a real-time analytics platform requires careful planning and execution. Here are some steps to ensure a successful implementation:
1. Define Clear Objectives
Set clear goals and objectives for what you want to achieve with real-time analytics. This could include improving customer experiences, optimizing operations, or enhancing decision-making processes.
2. Build a Skilled Team
Assemble a team with the necessary skills and expertise to manage the implementation. This team should include data analysts, IT professionals, and business stakeholders.
3. Develop a Data Strategy
Create a comprehensive data strategy that outlines how data will be collected, processed, and analyzed. Ensure data governance practices are in place to maintain data quality and integrity throughout the process.
4. Choose the Right Technology
Select a real-time analytics platform that meets your specific needs, considering factors such as integration capabilities, scalability, and user experience. Consider platforms that support robust data pipelines for efficient data flow and processing. Ensure that the platform aligns with your existing IT infrastructure and future growth plans.
5. Pilot the Solution
Before a full-scale rollout, conduct a pilot program to test the platform’s capabilities and performance in a controlled environment. This allows you to identify any potential issues and make necessary adjustments before widespread implementation.
6. Train Your Team
Provide comprehensive training for your team on how to use the new platform effectively. Ensure that users understand how to interpret data, create reports, and utilize advanced features.
7. Monitor and Optimize
After implementation, continuously monitor the performance of the analytics platform. Gather feedback from users and make iterative improvements to optimize its effectiveness and address any emerging needs.
8. Evaluate ROI
Regularly assess the return on investment from your real-time analytics solution. Measure improvements in decision-making, operational efficiency, and customer satisfaction to ensure that the platform is delivering the expected value.
Conclusion
Real-time analytics platforms are essential tools for modern businesses, offering the ability to process and analyze data instantly to drive timely decision-making and operational efficiency. As technology continues to advance, the capabilities of these platforms will expand, providing even greater insights and opportunities for businesses to stay ahead of the competition. By carefully selecting the right solution, considering key features, and implementing best practices, organizations can harness the power of real-time analytics to achieve their goals and thrive in an increasingly data-driven world.
For more insights on Analytics and its applications, read our blogs:
A Guide to Predictive, Prescriptive, Descriptive and Real Time Analytics
AI in Predictive Analytics Solutions: Unlocking Future Trends and Patters in the USA (2024 & Beyond)
Predictive Analytics Solutions for Business Growth in Georgia