Descriptive Analytics: Understanding the Past to Inform the Future
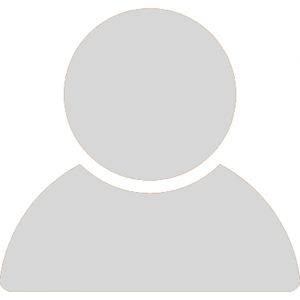
Audio : Listen to This Blog.
In the ever-evolving landscape of data analytics, businesses increasingly rely on data to make informed decisions, drive strategies, and optimize operations. How descriptive analytics can be applied within various organizations and how it works in providing insights and conclusions from raw data for informed decision-making is crucial for understanding its value. Among the various branches of analytics, descriptive analytics holds a foundational place, providing critical insights into historical data to paint a comprehensive picture of past performance. This blog delves into the significance of descriptive analytics, its methodologies, tools, and its crucial role in shaping future strategies.
Understanding Descriptive Analytics
What is Descriptive Analytics?
Descriptive analytics is the process of summarizing historical data to identify patterns, trends, and insights. It answers the question, “What happened?” by analyzing past data to understand the performance and behavior of various business aspects. Descriptive analytics can help in various business applications such as supply chain management, marketing campaign improvement, customer segmentation, operational efficiency analysis, and financial analysis. Unlike predictive analytics or prescriptive analytics, which focus on forecasting future trends and prescribing actions, descriptive analytics is retrospective, focusing solely on past data.
Key Components of Descriptive Analytics
Data Collection: Gathering relevant data from various sources such as transactional databases, logs, and external datasets is essential. This ensures the data is accurate, comprehensive, and representative of the subject being analyzed.
Data Cleaning: Ensuring data accuracy by identifying and correcting errors, inconsistencies, and missing values.
Data Aggregation: Combining data from different sources to create a comprehensive dataset.
Data Analysis: Using statistical methods and tools to analyze the data and identify patterns and trends.
Data Visualization: Presenting the analyzed data through charts, graphs, dashboards, and reports for easy interpretation.
Importance of Descriptive Analytics
Informing Decision Making
Descriptive analytics provides a factual basis for decision-making by offering a clear view of what has transpired in the past. Analyzing various data points such as social media engagement, email open rates, and number of subscribers can optimize marketing campaigns and understand the company’s performance. Businesses can use these insights to understand their strengths and weaknesses, make informed strategic decisions, and set realistic goals.
Performance Measurement Using Key Performance Indicators
Organizations use descriptive analytics to measure performance against key performance indicators (KPIs). By tracking metrics over time, businesses can assess their progress, identify areas for improvement, and make necessary adjustments to achieve their objectives.
Enhancing Customer Understanding with Historical Data
By analyzing historical customer data, businesses can gain valuable insights into customer behavior, preferences, and buying patterns. By analyzing historical sales data, businesses can identify patterns, seasonality, and long-term trends, which helps in decision-making and forecasting future performance. This information helps in creating targeted marketing strategies, improving customer service, and enhancing customer satisfaction.
Operational Efficiency
Descriptive analytics helps businesses optimize their operations by identifying inefficiencies and areas of waste. By understanding past performance, organizations can streamline processes, reduce costs, and improve productivity.
Methodologies in Descriptive Analytics
Data Mining
Data mining involves exploring large datasets to discover patterns, correlations, and anomalies. Exploratory data analysis involves techniques such as summary statistics and data visualization to understand data characteristics and identify initial patterns or trends. Techniques such as clustering, association rule mining, and anomaly detection are commonly used in descriptive analytics to uncover hidden insights.
Descriptive Statistics and Analysis
Statistical analysis uses mathematical techniques to analyze data and draw conclusions. Diagnostic analytics focuses on explaining why specific outcomes occurred and is used to make changes for the future. Descriptive statistics such as mean, median, mode, standard deviation, and variance provide a summary of the data’s central tendency and dispersion.
Data Visualization
Data visualization is a key aspect of descriptive analytics, enabling businesses to present complex data in an easily understandable format. Tools like bar charts, line graphs, pie charts, and histograms help in identifying trends and patterns visually.
Reporting
Reporting involves generating structured reports that summarize the analyzed data. These reports provide stakeholders with actionable insights and facilitate data-driven decision-making.
Tools for Descriptive Analytics
Microsoft Power BI
Power BI is a powerful business analytics tool that enables organizations to visualize their data and share insights across the organization. It offers robust data modeling, visualization, and reporting capabilities, making it a popular choice for descriptive analytics.
Tableau
Tableau is a leading data visualization tool that helps businesses create interactive and shareable dashboards. Its drag-and-drop interface and extensive visualization options make it easy to explore and present data effectively.
Google Data Studio
Google Data Studio is a free tool that allows users to create customizable and interactive reports. It integrates seamlessly with other Google services, making it a convenient choice for organizations using Google Analytics, Google Ads, and other Google products.
SAS Visual Analytics
SAS Visual Analytics offers a comprehensive suite of analytics tools for data exploration, visualization, and reporting. It leverages data science to transform raw data into understandable patterns, trends, and insights, enabling organizations to make informed decisions. It is known for its advanced analytics capabilities and user-friendly interface, catering to both novice and experienced users.
Qlik Sense
Qlik Sense is a self-service data visualization and discovery tool that empowers users to create personalized reports and dashboards. Its associative data model allows for intuitive data exploration and analysis.
Data Collection Methods
Effective descriptive analytics relies on accurate data collection methods, including:
Internal Databases: Leveraging data stored in company databases.
Customer Surveys: Collecting feedback directly from customers.
Website Analytics: Analyzing user behavior on company websites.
Social Media Data: Gathering insights from social media interactions and engagements.
Case Studies: Real-World Applications of Descriptive Analytics
Sales & Marketing
In sales and marketing, descriptive analytics can be used to analyze past sales data, identifying best-selling products, seasonal trends, and customer demographics. By transforming raw data into actionable insights, businesses can better understand their market and make informed decisions. This information helps tailor marketing campaigns for better targeting and improved ROI. For instance, a company might find that a certain product sells well among young adults during the summer, leading them to focus their marketing efforts on that demographic during that season.
Retail Industry
A leading retail chain used descriptive analytics to analyze sales data from its various stores. By identifying patterns in customer purchases, the company was able to optimize inventory levels, improve product placement, and increase sales. Descriptive analytics also helped the retailer segment its customer base and develop targeted marketing campaigns, resulting in higher customer engagement and loyalty.
Healthcare Sector
A healthcare provider utilized descriptive analytics to examine patient data and identify trends in disease outbreaks, treatment effectiveness, and patient outcomes. This analysis enabled the organization to improve patient care, streamline operations, and allocate resources more efficiently. By understanding historical data, the healthcare provider could also predict future healthcare needs and plan accordingly.
Financial Services
A financial institution leveraged descriptive analytics to analyze transaction data and detect fraudulent activities. By identifying unusual patterns and anomalies, the bank could prevent fraud and enhance its security measures. Additionally, descriptive analytics helped the bank understand customer behavior, enabling it to offer personalized financial products and services.
Manufacturing Industry
A manufacturing company used descriptive analytics to monitor production processes and identify inefficiencies. By analyzing machine performance data, the company could predict maintenance needs, reduce downtime, and improve overall productivity. Descriptive analytics also helped the manufacturer optimize supply chain operations and reduce operational costs.
Human Resources
In HR, descriptive analytics can identify top performers, track employee turnover rates, and improve talent acquisition strategies. For example, by analyzing employee data, a company might find that turnover is highest among new hires within the first six months. This insight can lead to improved onboarding processes and retention strategies.
Best Practices for Implementing Descriptive Analytics
Define Clear Objectives
Before embarking on a descriptive analytics initiative, it is crucial to define clear objectives. Understanding what you want to achieve with your analysis will guide the data collection, analysis, and reporting processes.
Ensure Data Quality
High-quality data is the foundation of effective descriptive analytics. Invest in data cleaning and validation processes to ensure the accuracy, consistency, and completeness of your data.
Choose the Right Tools
Selecting the appropriate tools for data analysis and visualization is essential. Consider factors such as ease of use, scalability, integration capabilities, and cost when choosing analytics tools.
Focus on Visualization
Effective data visualization makes it easier to interpret and communicate insights. Invest in tools and techniques that allow you to create clear, interactive, and compelling visualizations.
Foster a Data-Driven Culture
Encourage a data-driven culture within your organization by promoting the use of data in decision-making. Provide training and resources to help employees develop their data literacy skills.
Regularly Review and Update Your Analysis
Descriptive analytics is an ongoing process. Regularly review and update your analysis to reflect new data and changing business conditions. Continuously seek feedback and make improvements to your analytics processes.
The Future of Descriptive Analytics
As technology advances and the volume of data continues to grow, the future of descriptive analytics looks promising. Here are some trends to watch:
Integration with Predictive and Prescriptive Analytics
Descriptive analytics will increasingly integrate with advanced analytics techniques such as predictive and prescriptive analytics. Predictive analytics makes predictions about future performance based on statistics and modeling, benefiting companies by identifying inefficiencies and forecasting future trends. This integration will provide a more comprehensive view of the data, enabling businesses to move from understanding the past to predicting and shaping the future.
Real-Time Analytics
The demand for real-time insights is growing. Future developments in descriptive analytics will focus on real-time data processing and analysis, allowing businesses to make timely and informed decisions.
AI and Machine Learning
Artificial intelligence (AI) and machine learning will play a significant role in enhancing descriptive analytics. These technologies will automate data analysis, uncover deeper insights, and provide more accurate and actionable recommendations.
Enhanced Data Visualization
Advancements in data visualization tools will enable more sophisticated and interactive visualizations. Businesses will be able to explore their data in new ways, uncover hidden patterns, and communicate insights more effectively.
Increased Accessibility
As analytics tools become more user-friendly and affordable, descriptive analytics will become accessible to a broader range of users. Small and medium-sized businesses will increasingly leverage descriptive analytics to gain a competitive edge.
Conclusion
Descriptive analytics is a vital component of any data-driven strategy. By providing a clear understanding of past performance, it empowers businesses to make informed decisions, optimize operations, and enhance customer experiences. As technology evolves, the capabilities of descriptive analytics will continue to expand, offering even greater insights and opportunities. By embracing descriptive analytics, organizations can build a solid foundation for future success, leveraging historical data to navigate the complexities of the modern business landscape.
For more insights on Analytics and its applications, read our blogs:
AI in Predictive Analytics Solutions: Unlocking Future Trends and Patters in the USA (2024 & Beyond)
Predictive Analytics Solutions for Business Growth in Georgia
Prescriptive Analytics: Definitions, Tools, and Techniques for Better Decision Making